Publications by Tags
Selected Papers Adaptation, Benchmarks, Mathematics, Robustness, Safety,Selected Papers
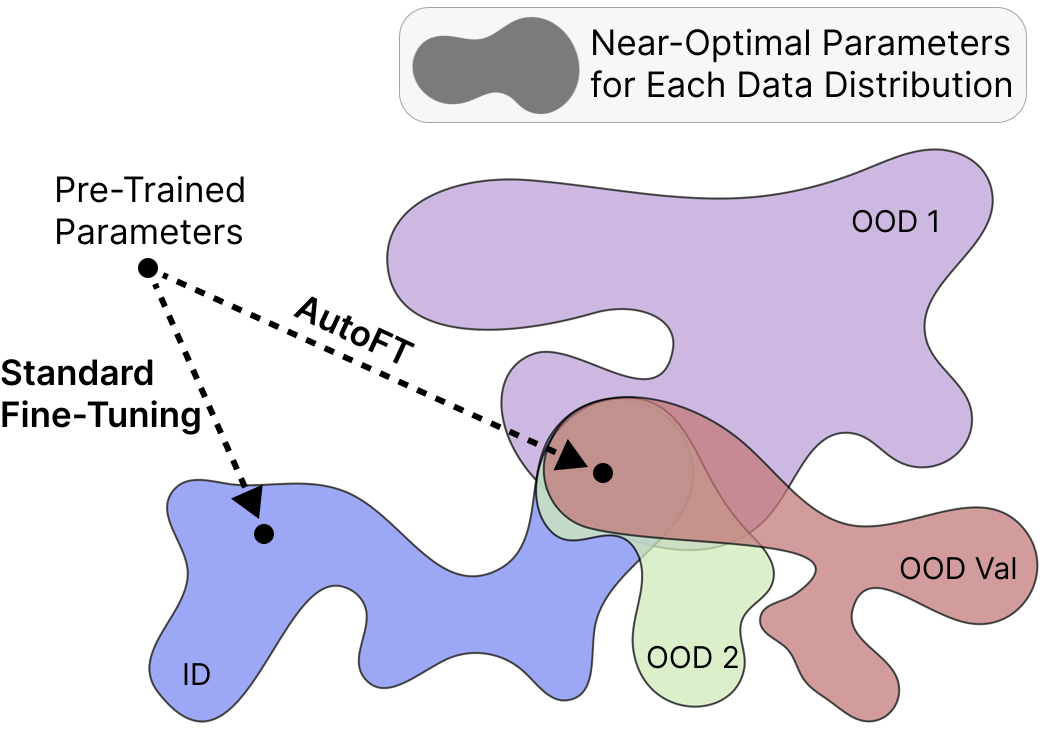
Caroline Choi*, Yoonho Lee*, Annie Chen, Allan Zhou, Aditi Raghunathan, Chelsea Finn
PreprintAutoFT is a data-driven approach to robust fine-tuning for foundation models. AutoFT searches for a robust fine-tuning procedure for a given task by optimizing hyperparameters on a small OOD validation dataset. AutoFT attains new state-of-the-art performance on the ImageNet, WILDS-iWildCam, and WILDS-FMoW distribution shift benchmarks.
Selected Papers Adaptation, Robustness,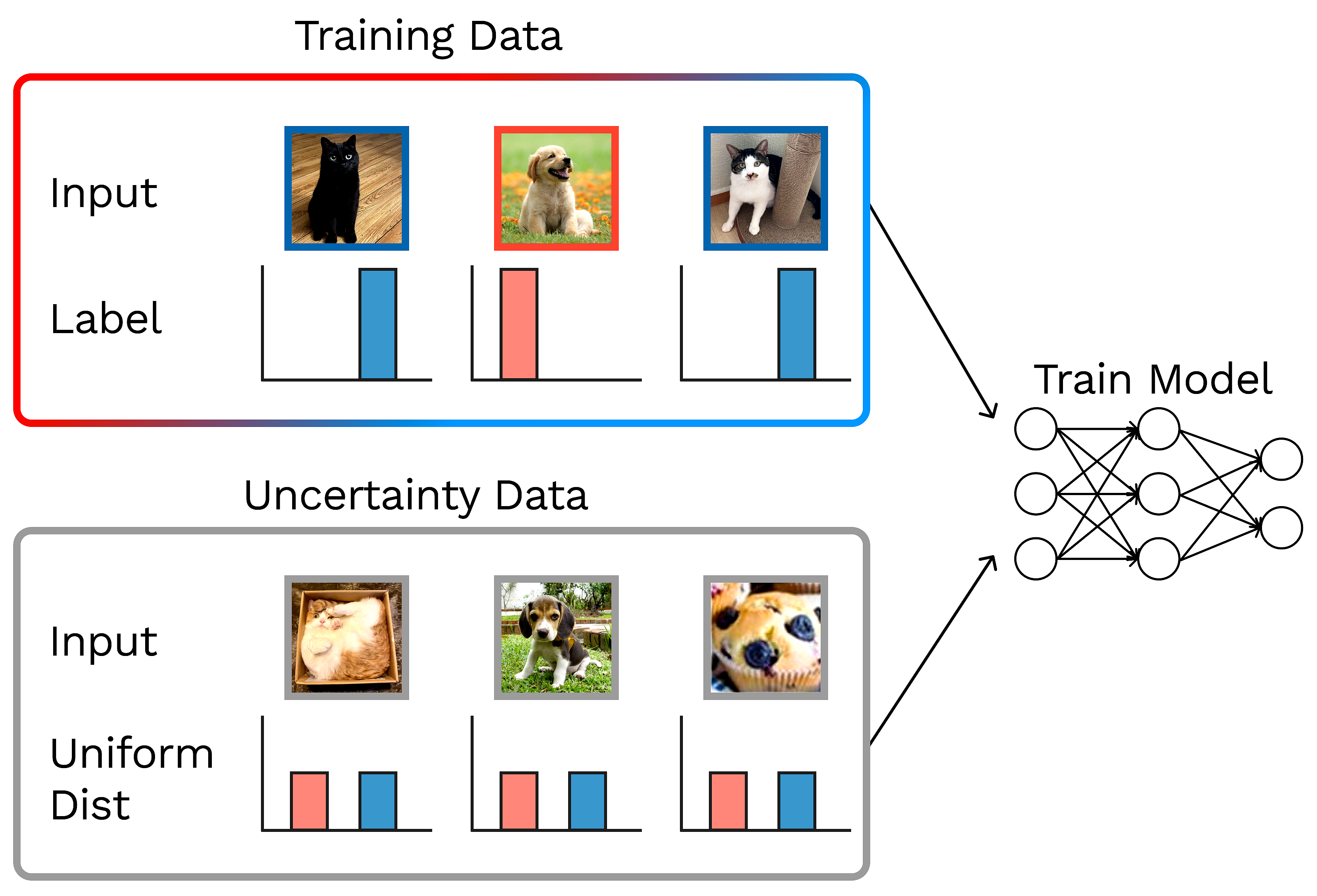
Caroline Choi*, Fahim Tajwar*, Yoonho Lee*, Huaxiu Yao, Ananya Kumar, Chelsea Finn
ICLR 2023 Workshops: TrustML, ME-FOMOData-Driven Confidence Minimization (DCM) is a framework for training models to make conservative predictions in safety-critical settings. We find that confidence minimization on a good choice of uncertainty dataset provably detects out-of-distribution examples and also yields improvements for selective classification.
Selected Papers Robustness, Safety,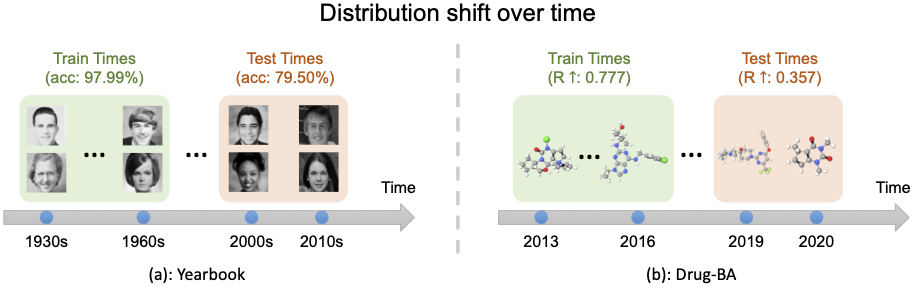
Huaxiu Yao*, Caroline Choi*, Bochuan Cao, Yoonho Lee, Pang Wei Koh, Chelsea Finn
NeurIPS 2022Wild-Time is a benchmark for real-world distribution shifts over time. We curated five datasets that exhibit temporal shift and span diverse applications and data modalities. We benchmark methods from the continual learning and domain generalization literature and find that no approach consistently outperforms ERM.
Selected Papers Benchmarks, Robustness,Adaptation
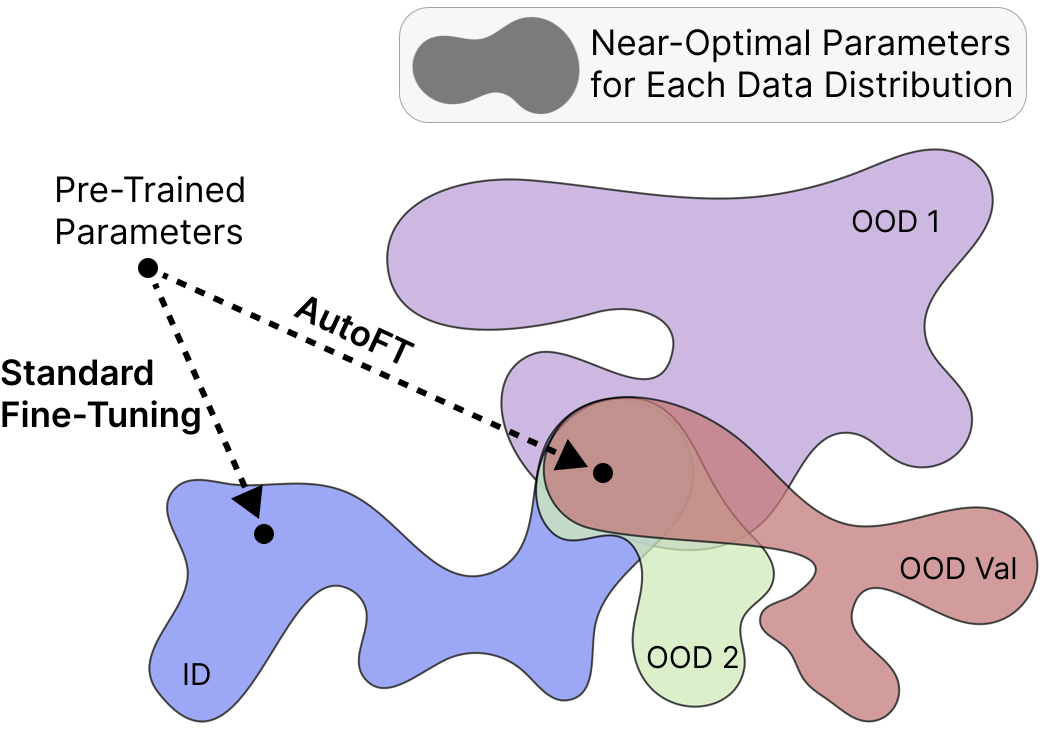
Caroline Choi*, Yoonho Lee*, Annie Chen, Allan Zhou, Aditi Raghunathan, Chelsea Finn
PreprintAutoFT is a data-driven approach to robust fine-tuning for foundation models. AutoFT searches for a robust fine-tuning procedure for a given task by optimizing hyperparameters on a small OOD validation dataset. AutoFT attains new state-of-the-art performance on the ImageNet, WILDS-iWildCam, and WILDS-FMoW distribution shift benchmarks.
Selected Papers Adaptation, Robustness,Benchmarks
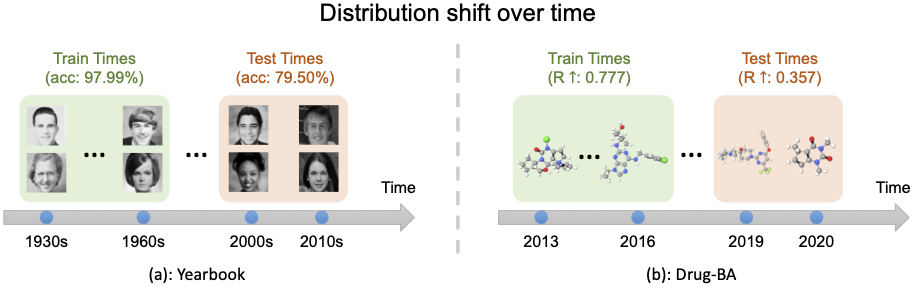
Huaxiu Yao*, Caroline Choi*, Bochuan Cao, Yoonho Lee, Pang Wei Koh, Chelsea Finn
NeurIPS 2022Wild-Time is a benchmark for real-world distribution shifts over time. We curated five datasets that exhibit temporal shift and span diverse applications and data modalities. We benchmark methods from the continual learning and domain generalization literature and find that no approach consistently outperforms ERM.
Selected Papers Benchmarks, Robustness,Mathematics
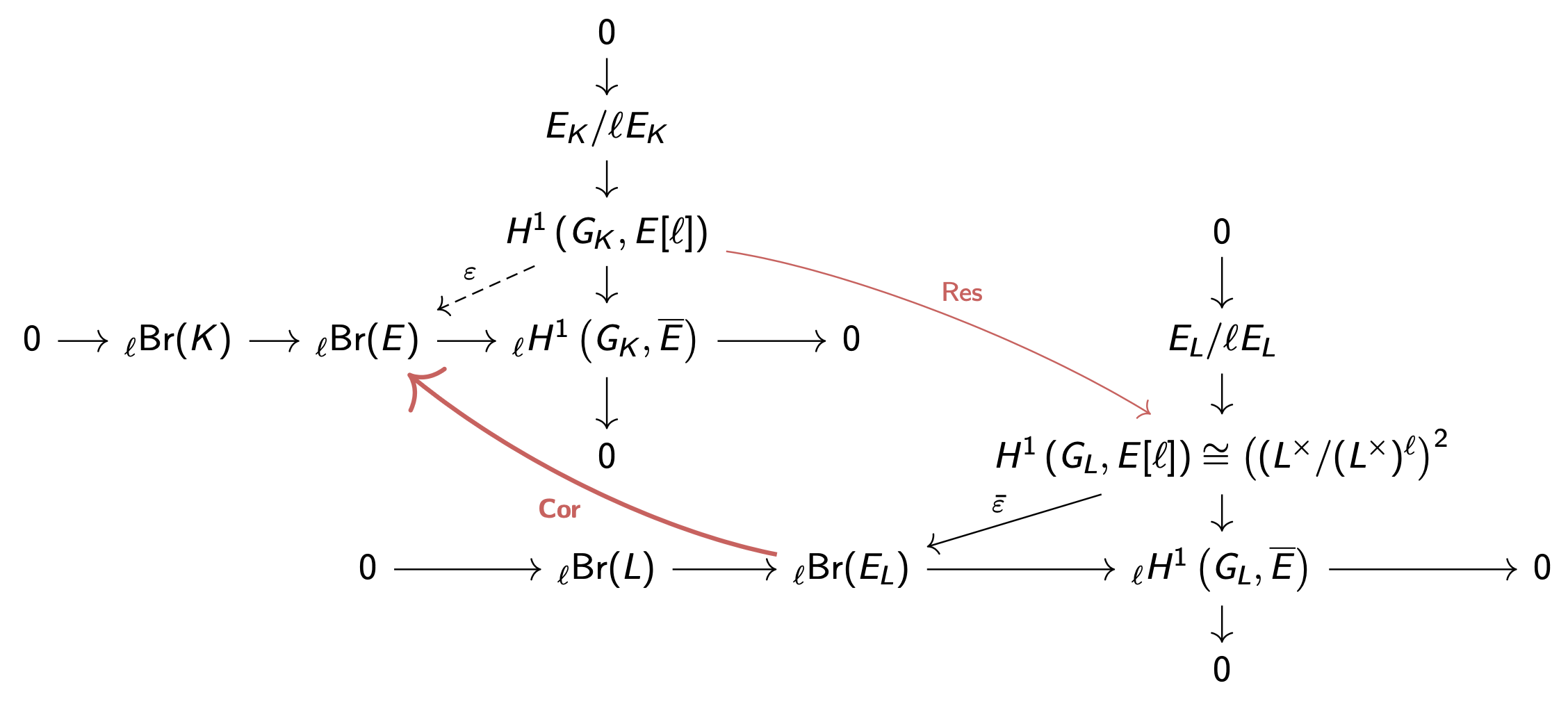
Mateo Attanasio*, Caroline Choi*, Andrei Mandelshtam*, Charlotte Ure*
Proceedings of the American Mathematical SocietyThe Brauer group is a tool that helps us understand the shape and characteristics of a mathematical space, known as a variety. We study Brauer groups of elliptic curves and improve bounds on a measure of their complexity, known as symbol length.
Mathematics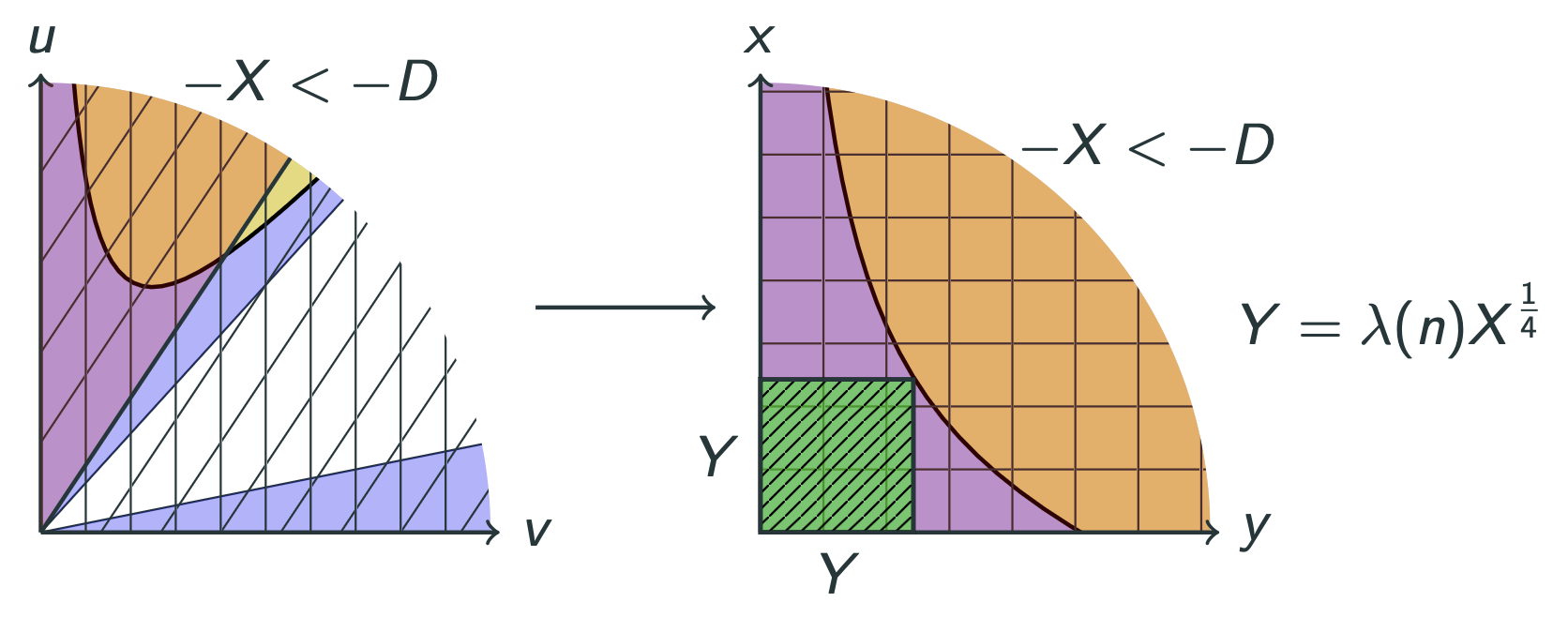
Talia Blum*, Caroline Choi*, Alexandra Hoey*, Jonas Iskander*, Kaya Lakein*, Thomas Martinez*
Transactions of the American Mathematical SocietyWe prove new bounds on the class number, first conjectured to exist by Gauss in 1801. We leverage connections between number theory and algebraic geometry, and construct a new family of maps between ideal class groups and elliptic curves.
Mathematics
Aman Agrawal*, Caroline Choi*, Nathan Sun*
Annals of CombinatoricsWe proved a "stabilization" conjecture by Gunnells et al. (2019) arising in the study of new permutation statistics known as weights. We prove this conjecture, and further show that permutation weights exhibit a recurrence relation.
MathematicsRobustness
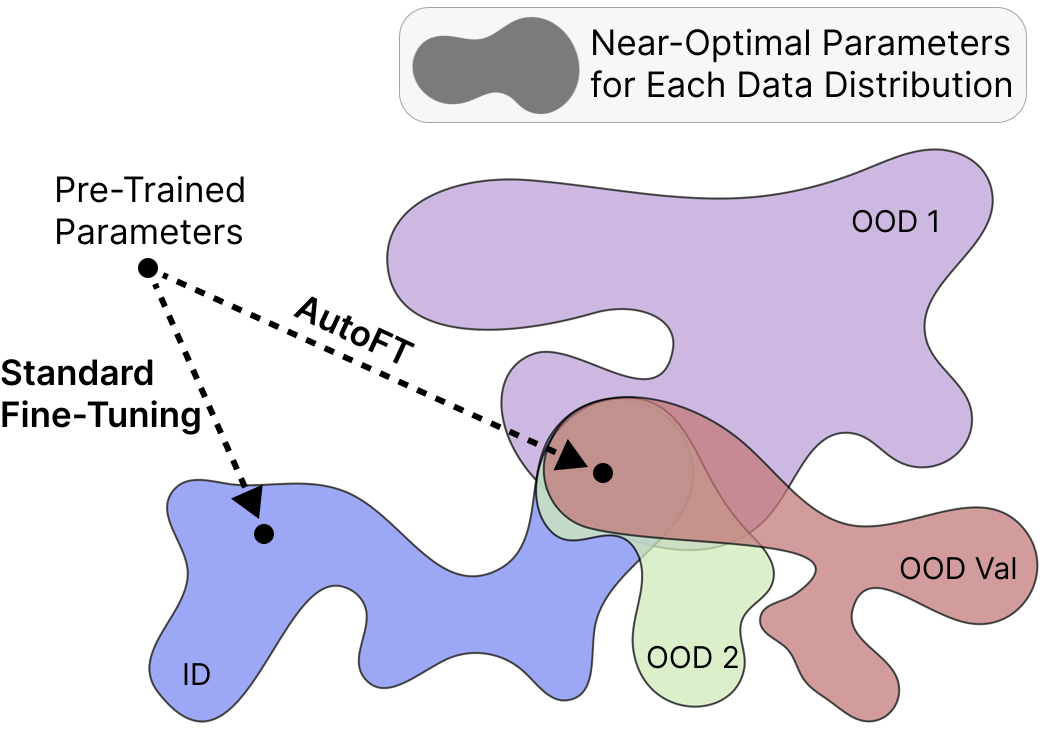
Caroline Choi*, Yoonho Lee*, Annie Chen, Allan Zhou, Aditi Raghunathan, Chelsea Finn
PreprintAutoFT is a data-driven approach to robust fine-tuning for foundation models. AutoFT searches for a robust fine-tuning procedure for a given task by optimizing hyperparameters on a small OOD validation dataset. AutoFT attains new state-of-the-art performance on the ImageNet, WILDS-iWildCam, and WILDS-FMoW distribution shift benchmarks.
Selected Papers Adaptation, Robustness,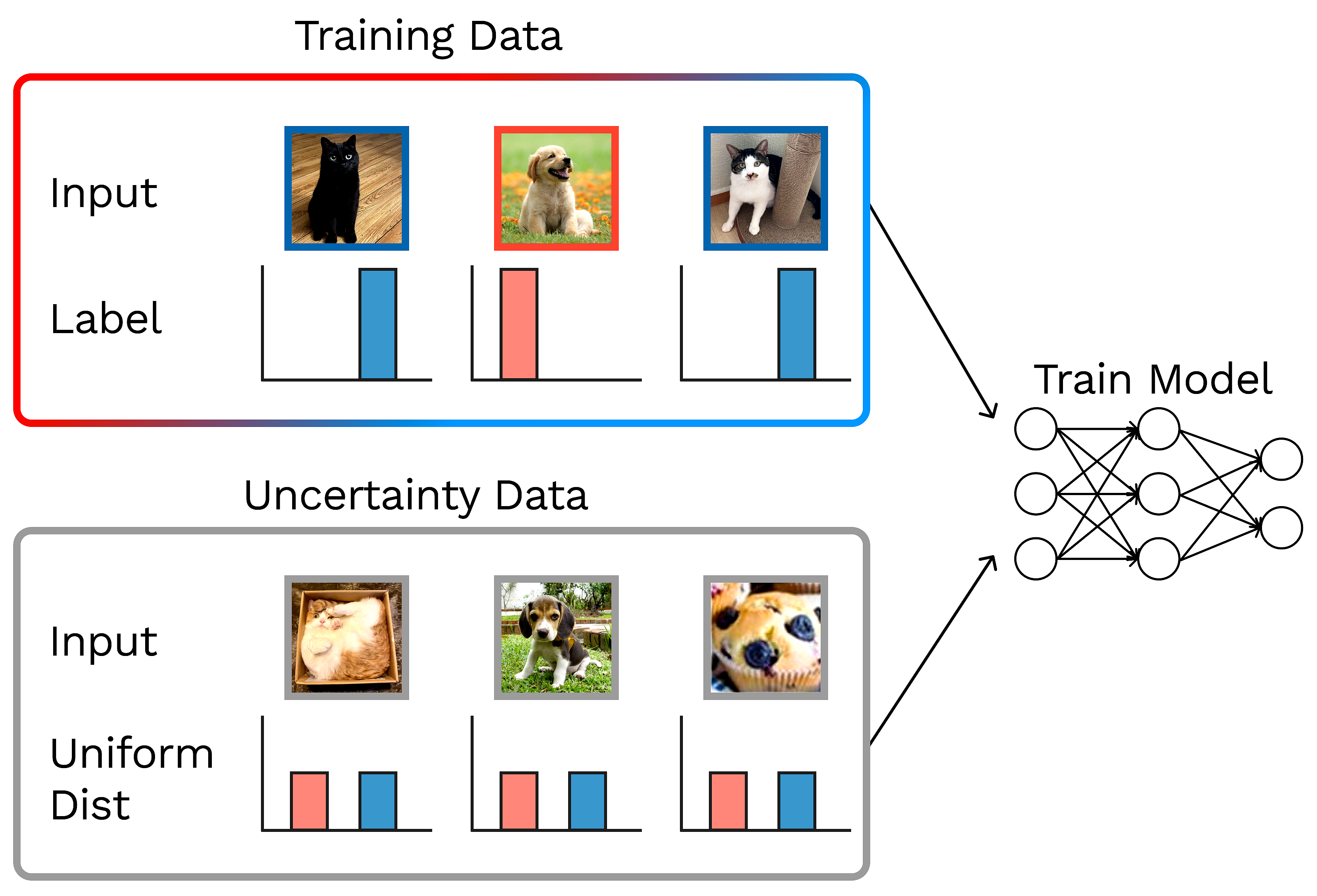
Caroline Choi*, Fahim Tajwar*, Yoonho Lee*, Huaxiu Yao, Ananya Kumar, Chelsea Finn
ICLR 2023 Workshops: TrustML, ME-FOMOData-Driven Confidence Minimization (DCM) is a framework for training models to make conservative predictions in safety-critical settings. We find that confidence minimization on a good choice of uncertainty dataset provably detects out-of-distribution examples and also yields improvements for selective classification.
Selected Papers Robustness, Safety,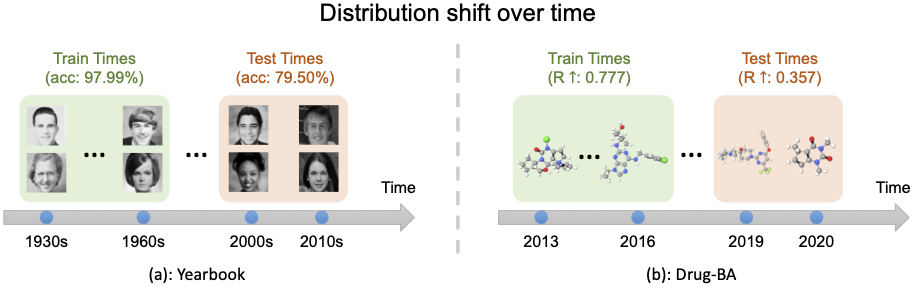
Huaxiu Yao*, Caroline Choi*, Bochuan Cao, Yoonho Lee, Pang Wei Koh, Chelsea Finn
NeurIPS 2022Wild-Time is a benchmark for real-world distribution shifts over time. We curated five datasets that exhibit temporal shift and span diverse applications and data modalities. We benchmark methods from the continual learning and domain generalization literature and find that no approach consistently outperforms ERM.
Selected Papers Benchmarks, Robustness,Safety
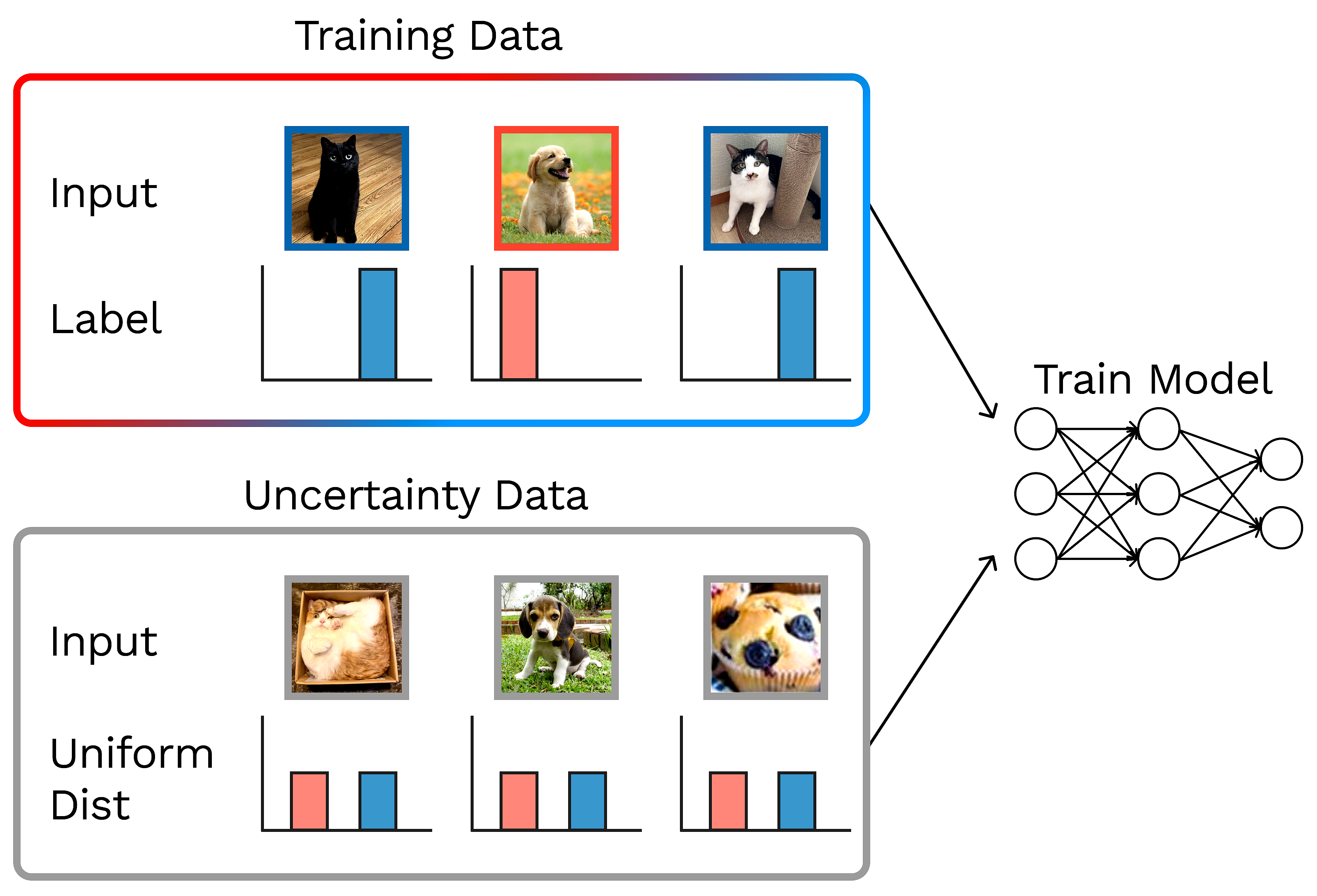
Caroline Choi*, Fahim Tajwar*, Yoonho Lee*, Huaxiu Yao, Ananya Kumar, Chelsea Finn
ICLR 2023 Workshops: TrustML, ME-FOMOData-Driven Confidence Minimization (DCM) is a framework for training models to make conservative predictions in safety-critical settings. We find that confidence minimization on a good choice of uncertainty dataset provably detects out-of-distribution examples and also yields improvements for selective classification.
Selected Papers Robustness, Safety,